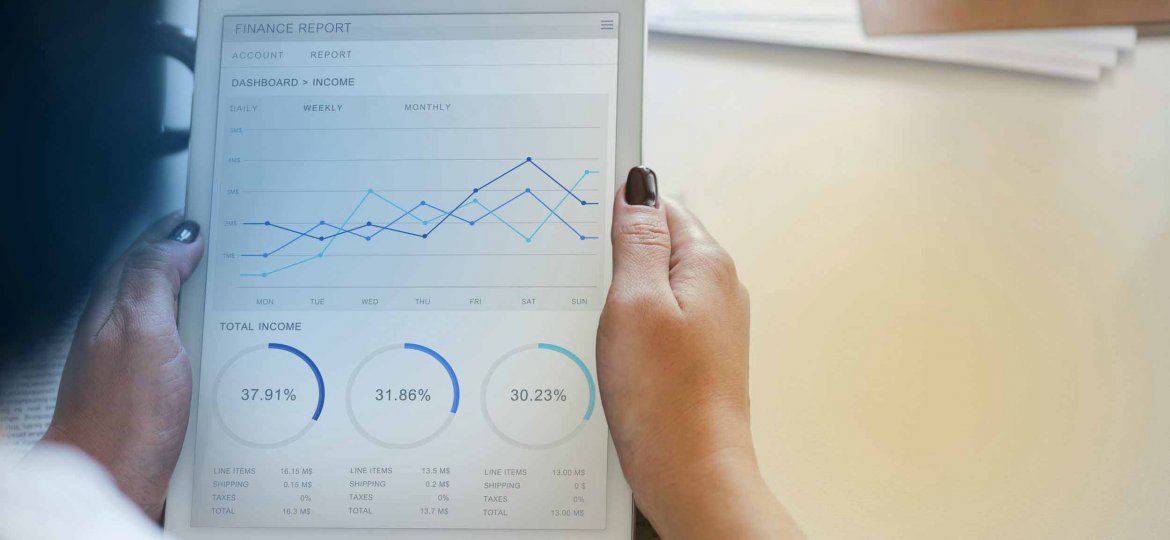
What is Business Intelligence(BI)?
Business Intelligence(BI) is the set of methodologies, processes, architectures, and technologies that transform raw data into meaningful and useful information used to enable more effective strategic, tactical, and operational insights and decision-making, OR one can simply say getting the right information to the right people at the right time.
BI is much more than a set of dashboards and reports. In facts, the technology of BI is only the tip of the iceberg. The real substance behind BI lies in how different parts of an organization collect, share, and process data. BI is best to utilize as a service to the entire organization, with each business area playing a role in its upkeep and improvement. Most Organization underestimate BI as simply an afterthought or bolt-on to their operational software applications. But the truth is a good BI is critical because it provides visibility into what is happening within those applications and amongst its people. BI is actually the one programme in any organization that can touch virtually every area of the business. Artificial Intelligence (AI) and Machine Learning(ML) are the heart of digital transformation by enabling organizations to exploit their growing volume wealth of Big Data to optimize key business and operational use cases.
The Evolutionary Path of BI
- The first generation of BI: In the 1990s, It could take weeks of IT work and coding to create a series of highly formatted reports. At that time, companies embedded proprietary BI tools, such as Crystal Reports, into their desktop or client/server applications using proprietary application programming interfaces (APIs). Small discrepancies in the data ultimately impacted final figures, proving to be extremely costly and, as a result, the time spent checking the data a lot of company wasted resource, which could otherwise have been invested elsewhere in the business. In this generation of BI, businesses simply could not make snapshot reactive decisions as they can today.
- Second generation of BI : Embedding proprietary BI tools or using proprietary APIs started to change in the 2000s (during the second generation of BI), when the rise of both standardized data warehouses, in-memory engines and Web technologies made possible the access of large amounts of normalized data through intuitive drag-and-drop report- and dashboard-building tools, such as Power BI or Tableau. By doing so analysts and business users could finally self-serve their analysis without the involvement on IT personnel. This generation also included improved embedding techniques, enabling companies to create and integrate reports and dashboards inside applications using HTML, iFrames and SOAP-based Web services interfaces. After creating and integrating reports and dashboards employees could access more than a static report, and as dashboards grew in popularity, users were able to quickly investigate the data to help analysts and business users make better-informed business decisions across a variety of domains. Analysts and business users ultimately had to go looking for the data, rather than the other way around. So, this problem remained with this generation of BI.
- The third generation of BI: In order to solve the 2nd generation problem, brings us neatly to today, a world of increasingly multi-structured data sets that all need to be analyzed. Now a day businesses data are growing exponentially, but many of them are now recognizing the newfound responsibility of using data to create more value, whether it is to keep costs down, drive additional sales, engage customers more fully or improve process efficiency, such as if one’s competitor suddenly dropped price, how are others going to handle this situation and how can they make that decision if they can’t rapidly predict the impact to their profitability after a quarter or two.
The democratization of AI and ML
Democratization is defined as the action of making something accessible to everyone, to the “common masses”. Until recently, it requires a data scientist to write a code for any AI or ML algorithm in order to create a model that can predict the impact to one’s profitability before their next quarter so that they can also keep costs down, drive additional sales, engage customers more fully or improve process efficiency with maximum profit. But in this current era of increasing data volume, there is a shortage of qualified data scientists is often highlighted as one of the major handbrakes on the adoption of Big Data and AI. So, a growing number of tools are putting data scientists capabilities in hands of non-experts for better.
Transforming BI through AI and ML Democratization
Democratization has brought an explosion in the breadth and quality of self-service analytics platforms in recent years, which let non-technical employees tap the huge amounts of data businesses are sitting on. These platforms typically let users carry out simple, day-to-day analytic tasks—like creating reports or building data visualizations—rather than having to rely on the company’s data specialists. The employees using self-service analytics tool will output more than professional data scientists. Democratization is not just a simple analytic task that is being made more accessible, there are also a growing number of tools to help beginners start to build their own machine learning(ML) models, which comes with the pre-built algorithm and intuitive interfaces that make it easy for someone with little experience to get started. These tolls are aimed at developers rather than analysts and business users who use simpler self-service analytics platforms, but they mean it’s no longer necessary to have a Ph.D. in advanced statistics to get started.